Unpacking the technical fabric of AIM
In the rapidly advancing domain of digital marketing, precision and analytical depth are not just virtues but necessities. Our marketing mix modeling platform, AIM (Always-On Incremental Measurement), is engineered to meet these demands through its sophisticated fusion of hierarchical Bayesian models and dynamic nonlinear regression. This technical discourse provides a closer look at the statistical sophistication that powers AIM at a level that can resonate with both seasoned marketing analysts and data scientists.
Hierarchical Bayesian Models: Statistical Underpinnings
Hierarchical Bayesian models form the backbone of AIM, offering a statistical framework that adeptly handles the inherent complexity of marketing data. This approach is distinguished by its capacity to model data across multiple levels of hierarchy, acknowledging the nested structure that characterizes much of consumer data.
Bayesian Inference: Elevating Predictive Accuracy
Central to the hierarchical Bayesian approach is Bayesian inference, a process that iteratively updates the probabilities of hypotheses as new data emerges. This methodology employs Bayes’ theorem as a mathematical tool for updating the likelihood of our models’ parameters, based on the posterior distribution. This iterative process is particularly adept at refining model predictions in real-time, enhancing the predictive validity of marketing outcomes as the campaign unfolds.
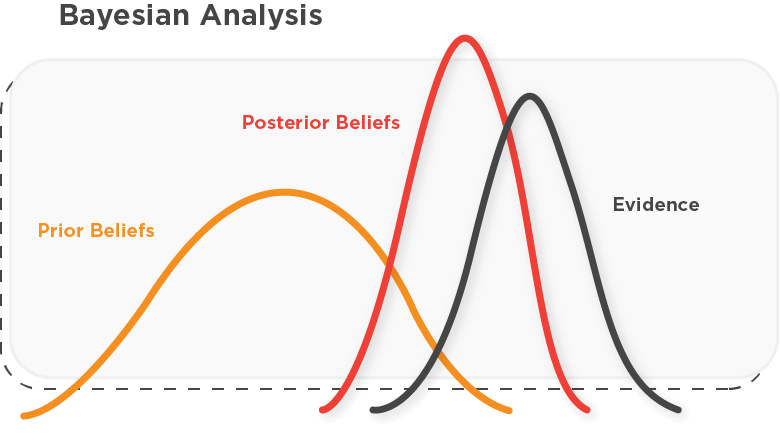
Operationalizing Hierarchical Models
The practical implementation of hierarchical Bayesian models in AIM leverages both the observed data and prior distributions to formulate a comprehensive view of the marketing landscape. This approach not only incorporates the variability observed within the data but also integrates expert knowledge and data from existing attribution platforms, even when that data is incomplete. Additionally, it includes insights gathered from questionnaires and historical incremental studies. The result is a single hybrid attribution algorithm uniquely tailored to your business, providing a nuanced and accurate understanding of marketing effectiveness.
AIM’s capacity to adjust for external influences—such as weather, national holidays, sporting events, and even pop concerts—on sales and conversions is a significant addition to this approach. For instance, a sudden heatwave can significantly boost sales of cooling products or beverages; AIM can predict these spikes by analyzing historical weather-related sales data. Similarly, national holidays or major sporting events can lead to changes in consumer buying patterns, which AIM anticipates by adjusting its models based on these events’ historical impacts. By accounting for these external factors, AIM provides a more nuanced and accurate understanding of the marketing dynamics at play.
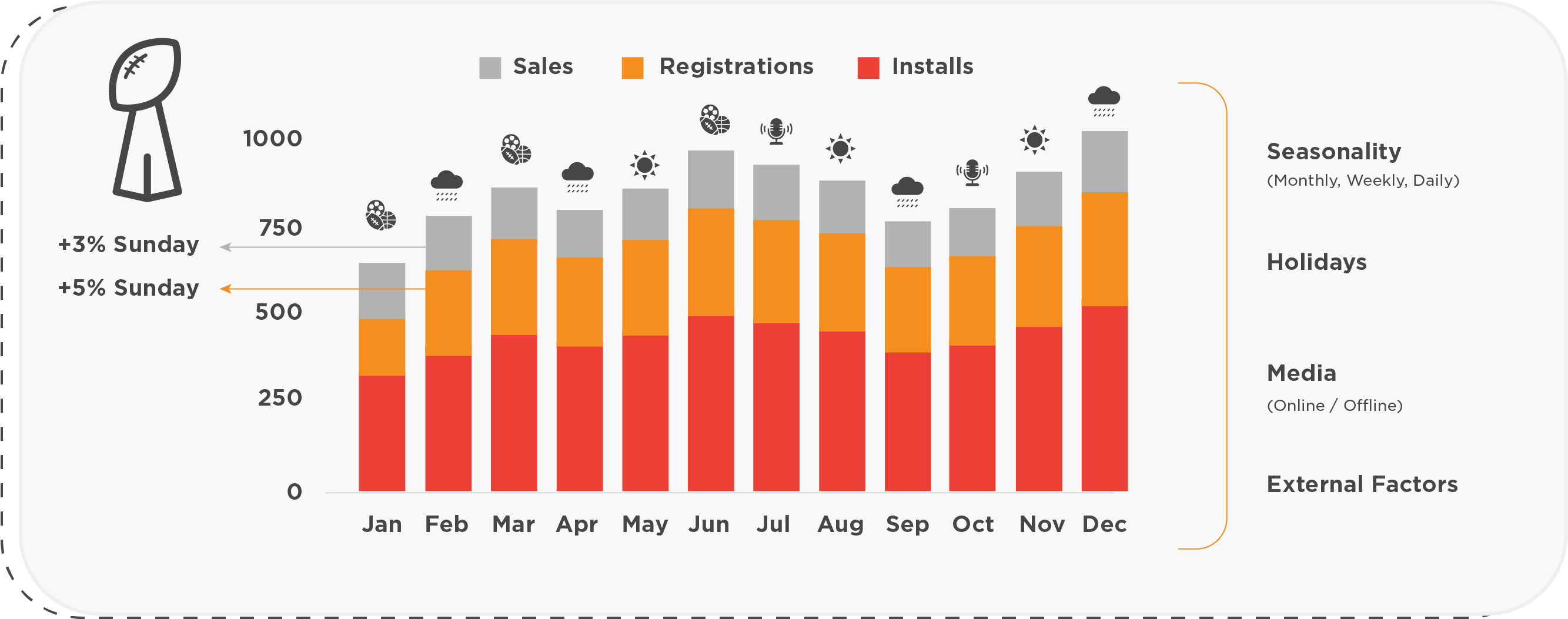
Dynamic Nonlinear Regression: The Complexities of Marketing Data
Dynamic nonlinear regression extends the capabilities of AIM by modeling the intricate and often nonlinear relationships inherent in marketing data. This approach is crucial for understanding how various inputs, such as marketing spend across different channels, translate into business outcomes. A key advantage of this dynamic feature is its ability to allow changes in creatives and network efficiency to propagate through to the parameter distributions faster than models that place all historical data into a single bucket. This rapid adaptation enhances the model’s responsiveness and accuracy, enabling more timely and effective marketing decisions.
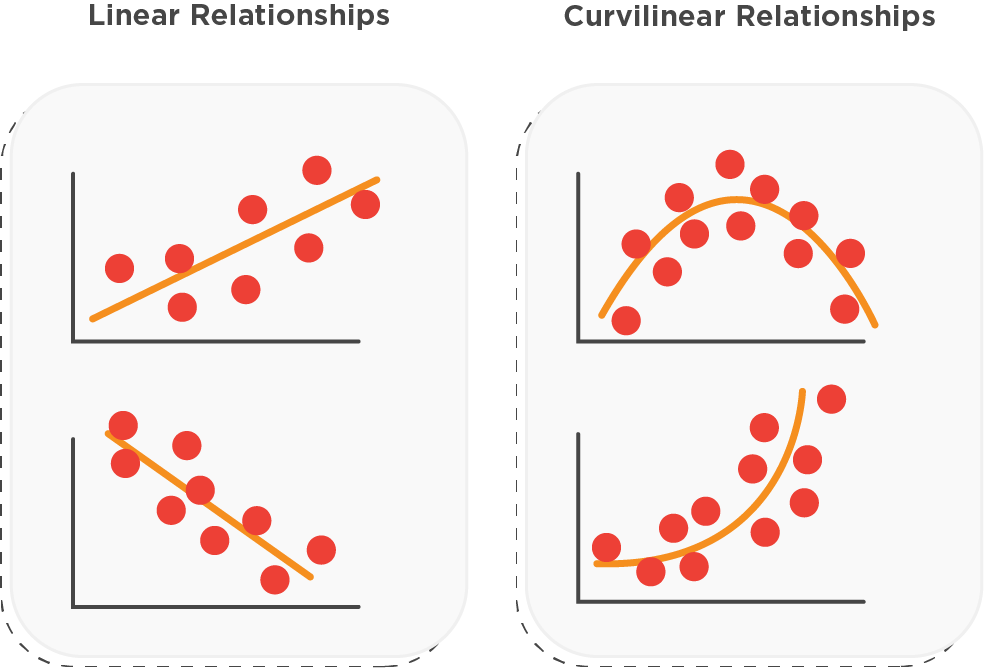
Modeling Nonlinear Interactions
The essence of dynamic nonlinear regression lies in its ability to capture the complex, dynamic interactions between marketing variables. By employing advanced mathematical functions, this technique maps out the curvilinear relationships that often exist between marketing inputs and outputs such as spend and sales.
Example: Nonlinear Dynamics in Digital Advertising
Consider the case where digital advertising spend exhibits a logarithmic relationship with sales, a common scenario in digital marketing. Initial increases in budget lead to significant sales uplifts; however, as spend continues to rise, incremental sales gains begin to diminish. AIM, through dynamic nonlinear regression, quantitatively models this relationship, identifying the point of diminishing returns to optimize advertising spend for maximum ROI.
Synthesizing Advanced Methodologies: The Core of AIM
The integration of hierarchical Bayesian models with dynamic nonlinear regression within AIM does not merely add to its analytical arsenal; it transforms AIM into a sophisticated predictive engine that navigates the complexities of the marketing world with precision. This synthesis enables AIM to offer nuanced insights into marketing effectiveness, forecasting the impact of various strategies with a level of accuracy and depth unmatched by traditional models.
Practical Insight: Strategizing with Precision
In multichannel marketing campaigns, AIM’s advanced methodology facilitates the strategic allocation of marketing budgets, timing of campaign launches, and optimization of channel mix. By modeling the interactive effects of various marketing initiatives and their nonlinear impact on sales, AIM empowers marketers to craft strategies that are not only data-driven but also aligned with the dynamic nature of consumer markets.
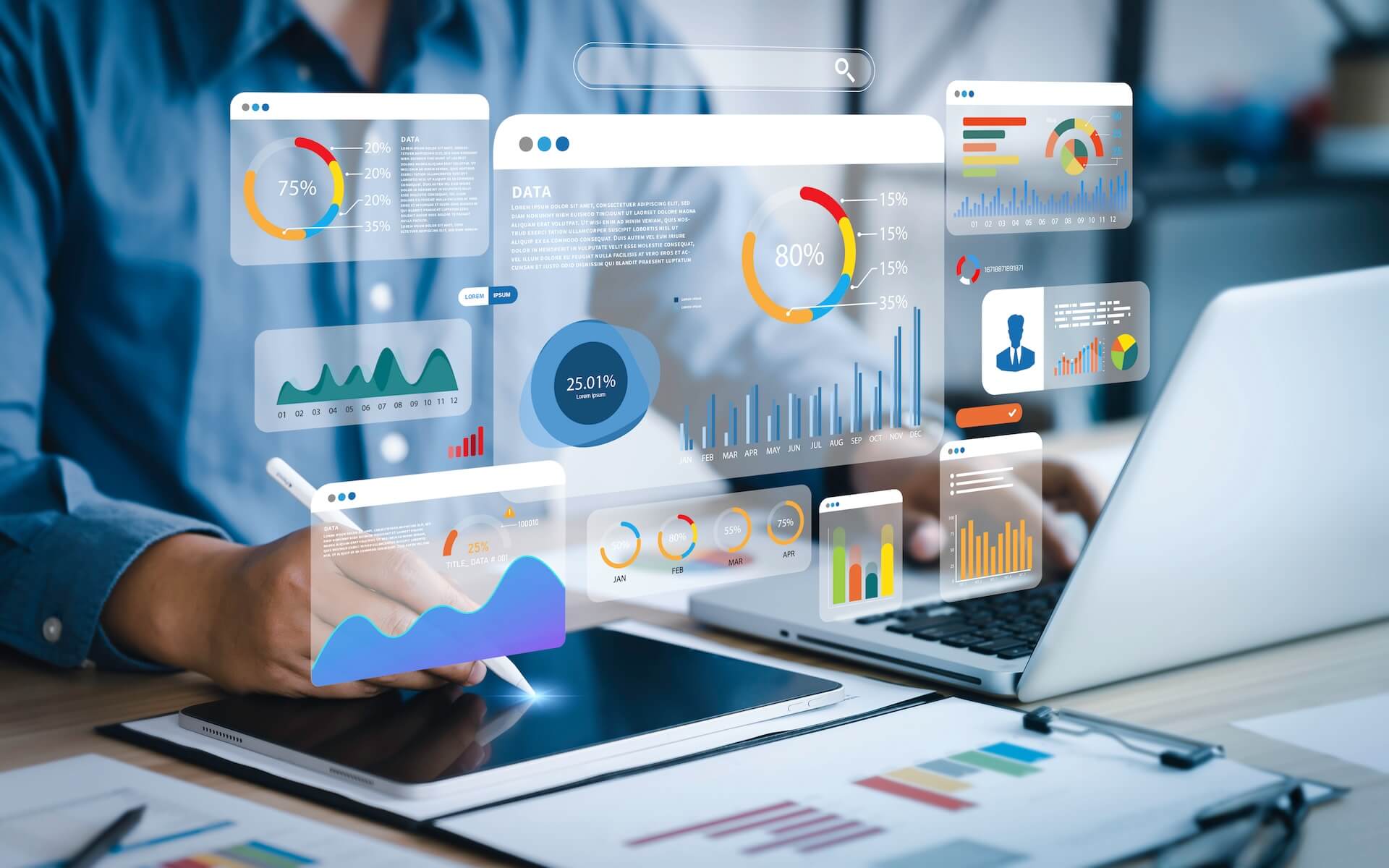
Incorporating the ability to adjust for external events and influences ensures that AIM’s predictions and recommendations remain relevant and precise, even in the face of unforeseen circumstances. This adaptability makes AIM an invaluable tool for marketers looking to navigate the complexities of the digital marketing landscape with confidence and precision.
Interested in exploring AIM in further detail? Contact us for a full consultation with our experts.