MMM can seem counterintuitive—but is your best compass for optimizing ROI
Marketing teams have long relied on last-touch attribution to measure and optimize their efforts. It’s simple and straightforward, allowing them to assign direct credit to the last marketing touchpoint leading to a conversion. But the simplicity of last-touch attribution is also its greatest weakness: It doesn’t give a holistic view of how various marketing channels and other external factors work together to drive results. That’s where marketing mix modeling (MMM) comes in.
If you’re new to marketing mix modeling, its output might seem counterintuitive at first glance. But there’s a method to what may seem like madness—rooted in advanced data science, complex algorithms, and a deeper understanding of how marketing truly works.
In this article, I’ll break down why MMM recommendations can seem counterintuitive and provide an analogy to help explain the logic behind this. Understanding why this happens will help you grasp MMM and make smarter, data-driven decisions.
How Does Marketing Mix Modeling Differ From Last-Touch Attribution?
MMM looks at a broader picture than last-touch attribution. Instead of focusing only on the last click or impression before a conversion, MMM analyzes how all your channels—digital and traditional—work together over time. It takes into account factors such as:
Seasonality
Does your target audience act differently during a specific time of year?
Diminishing Returns
Is a channel performing well but hitting the point where more spend won’t result in more conversions?
Ad Stock
How long do the effects of a particular ad last, and how should this affect your current spend?
External Factors
What market influences affect your business outcomes? Things like national holidays, major sporting events, or even the weather can impact how your marketing mix performs.
Media Source Interactions
How are interactions among your different media sources affecting overall performance? For instance, TV often interacts with search ads—viewers might see a TV commercial, then perform a search related to the featured product.
MMM captures all the complex dynamics a simpler attribution model might overlook.
These calculations produce actionable recommendations that may look illogical to an experienced marketer encountering MMM for the first time.
Why MMM Recommendations Can Seem Counterintuitive
Let’s say that you’ve set a cost-per-acquisition (CPA) target for your campaigns. After plugging your data into a platform such as AIM (Always-On Incremental Measurement) by Kochava, the system gives you recommendations on where to allocate your budget. But here’s the twist: It might recommend increasing spend on a channel with high CPA and reducing spend on a channel with low CPA.
Wait… what??? Why?
The MMM engine is doing something more sophisticated than simply comparing CPA across channels. It’s optimizing budget based on your entire marketing mix, taking into account factors including cost curves, seasonality, and diminishing returns.
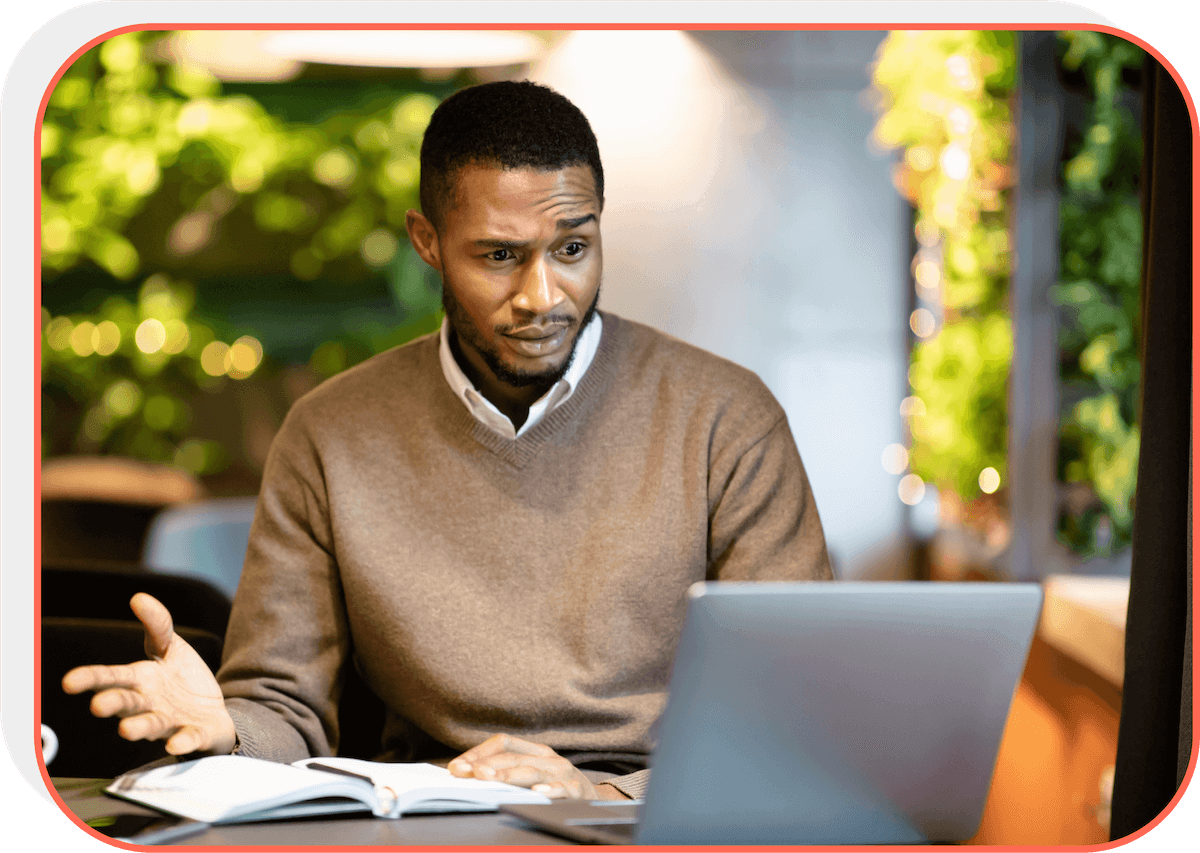
Using IronSource and Google Ads as examples:
- A high CPA on Google Ads might still be more efficient than a low CPA on IronSource, depending on where each channel sits on its cost curve. If Google still generates returns even with a high CPA, while IronSource has reached a point of diminishing returns, then increasing Google’s budget might give you better overall returns.
- Diminishing returns: Just because a channel has a low CPA (IronSource) doesn’t mean it can infinitely deliver at the same cost. If you pump more money into the channel, cost per acquisition might rise significantly, whereas a channel with higher CPA (Google) might still have room to scale up.
Understanding the Cost Curve: A Deeper Dive
To help you understand why MMM might recommend decreasing spend on a network with a lower CPA while increasing spend on another with a higher CPA, let’s break down the concept of cost curves and why they’re crucial for making optimal decisions. Once again, we’ll use IronSource and Google Ads as an example.
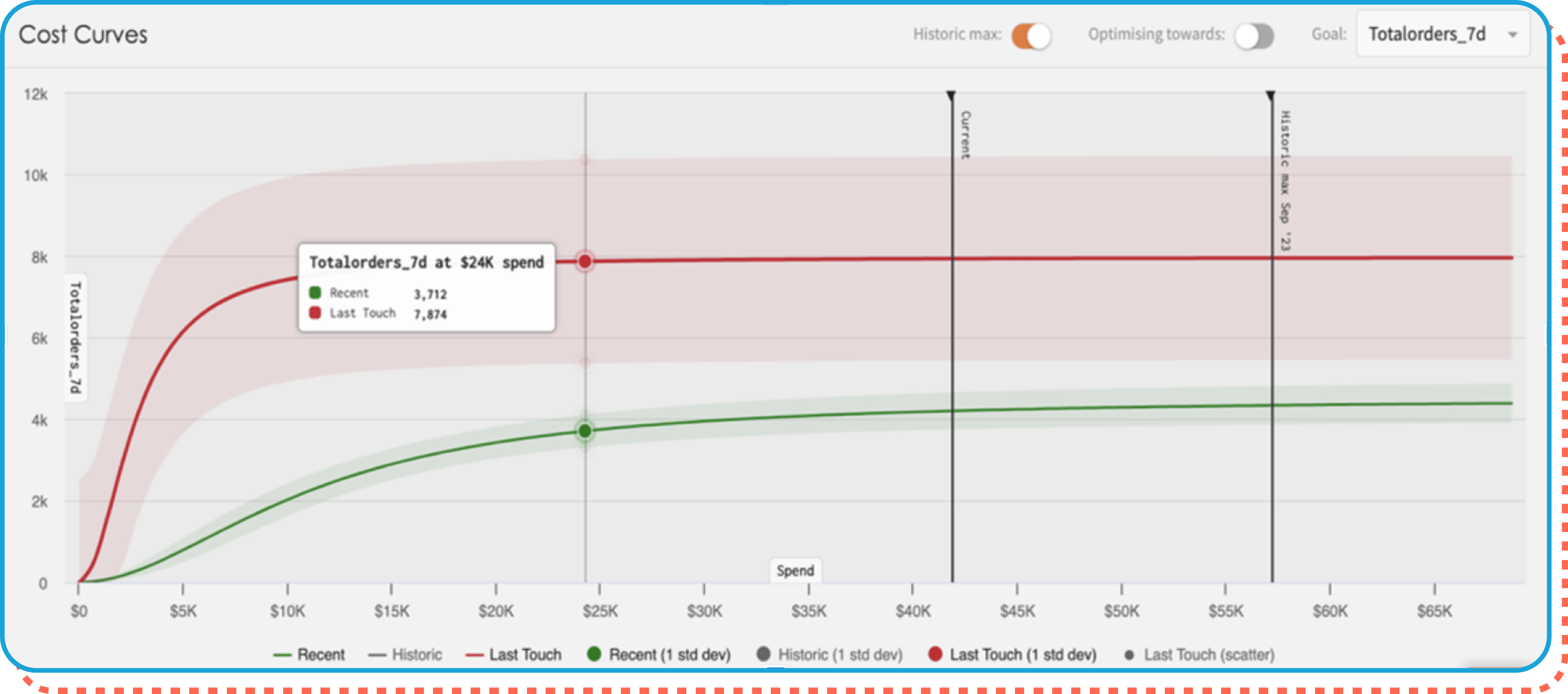
A last-touch attribution system doesn’t consider cost curves. This means it looks at only a simple average over time. Let’s say your CPA target is $10, and IronSource reports that over a month, it has delivered acquisitions at exactly $10. On the surface, this appears to meet your expectations.
However, what last-touch attribution doesn’t show you is that IronSource may have delivered 80 of these acquisitions at $6.25 each but another 20 at much higher costs—bringing the overall CPA to $10. Those higher-cost acquisitions often happen once you reach a point of diminishing returns, meaning that the early part of your budget is spent efficiently, but further investment yields much lower returns.
Here’s the breakdown:
- The first $500 spent on IronSource may deliver 80 acquisitions at $6.25 each, which is extremely efficient.
- The next $500 spent may deliver only 20 acquisitions, and at $25 each—far above your target CPA.
So, while the average CPA over the month seems to hit your target of $10, in reality, you’ve hit diminishing returns—and further spend on IronSource won’t be efficient.
This is exactly where an MMM system comes in. An MMM model doesn’t look at just averages; it calculates the marginal returns on every dollar spent. The system understands the performance curve of each channel and how to maximize return on your total budget.
In the case of IronSource:
- The MMM model recognizes that the first portion of your spend is highly efficient, delivering results well below your CPA target.
- Once you’ve hit the point of diminishing returns, however, the model realizes that continuing to spend more on IronSource will result in escalating costs without delivering additional value.
Now, the key insight from MMM is this: It may recommend spending 10% less on IronSource and reallocating it to Google Ads. Even though Google might have a higher CPA at first glance, the model might calculate that it can still deliver consistent results and, at a certain spend level, provide far greater return on investment compared to IronSource.
By redistributing that 10%:
- IronSource remains efficient, hitting its sweet spot without overspending.
- Google Ads, despite its seemingly higher CPA, is tapped into its broader scale, enabling you to drive more acquisitions without hitting a hard point of diminishing returns.
In essence, MMM calculates how to allocate your budget across channels to achieve maximum ROI, balancing efficiency with scalability. The model ensures that you’re not just meeting your CPA target—you’re getting the best possible return from every dollar spent across your entire marketing mix.
Analogy: Choosing the Best Car for a Road Trip
Let me offer an analogy that might make this clearer:
Imagine you’re going on a long road trip and have two cars, Car A and Car B. Car A gets 30 miles per gallon (mpg), while Car B gets 50 mpg. At first glance, you might think Car B is the better choice because it’s more fuel-efficient, just as you might think a marketing channel with a lower CPA is always the best.
But here’s the catch: Car A has a larger fuel tank and can drive much farther without needing to stop for gas. Car B, although more efficient, has a smaller tank and requires frequent stops to refuel.
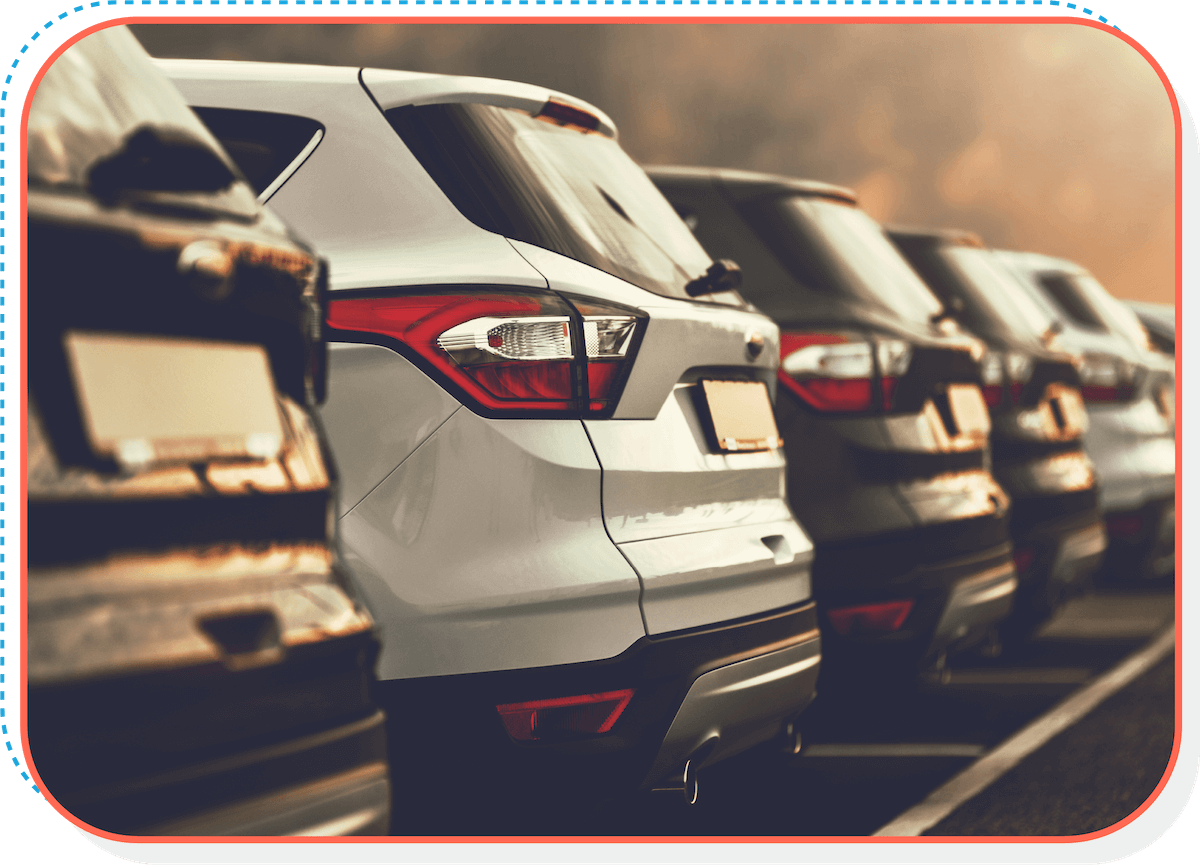
Now, your goal is to reach your destination as quickly as possible, and stopping frequently to refuel will slow you down. In this scenario, Car A—even though it burns more fuel per mile—can get you to your destination faster because it can go farther before stopping.
In this analogy:
- Car A represents a marketing channel with a higher CPA but much more scale.
- Car B represents a channel with a lower CPA but limited scale.
MMM helps you choose the option that gets you to your “destination”—your marketing goals—most efficiently, even if it means spending more on a channel with a higher CPA.
Trust the Data Science Behind the Recommendations
When marketing mix modeling offers a recommendation that seems counterintuitive—like increasing spend on a high-CPA channel or reducing spend on a low-CPA one—it’s because the system is processing far more variables than a human operator could possibly consider.
MMM takes into account a massive volume of data points that interact in complex ways. For instance, the system evaluates performance metrics over time, understanding seasonality, managing the interplay among media sources, and predicting how each additional dollar spent impacts performance. No human can process all of these variables simultaneously.
A human might be able to look at a few metrics—like CPA or total spend—across a couple of channels and make adjustments. But MMM incorporates thousands of data points, from cost curves and external factors to media interactions and diminishing returns, to produce its optimization.
MMM helps you manage not only your performance goals, like hitting your CPA target, but also how best to allocate a fixed budget. For instance, if your goal is to spend a million dollars per month, AIM shows you the best way to allocate that spend, even if it means you won’t hit your target CPA perfectly. Alternatively, if your goal is to hit a specific CPA and you program the system for that, AIM may recommend spending less than your million-dollar budget to meet that CPA target. The system is designed to balance your goals, giving you the most efficient way to use your resources.
In short, MMM makes recommendations that go far beyond human capacity for manual optimization, incorporating multiple, overlapping layers of information to aid your decision making.
Final Thoughts: Embracing the Power of MMM
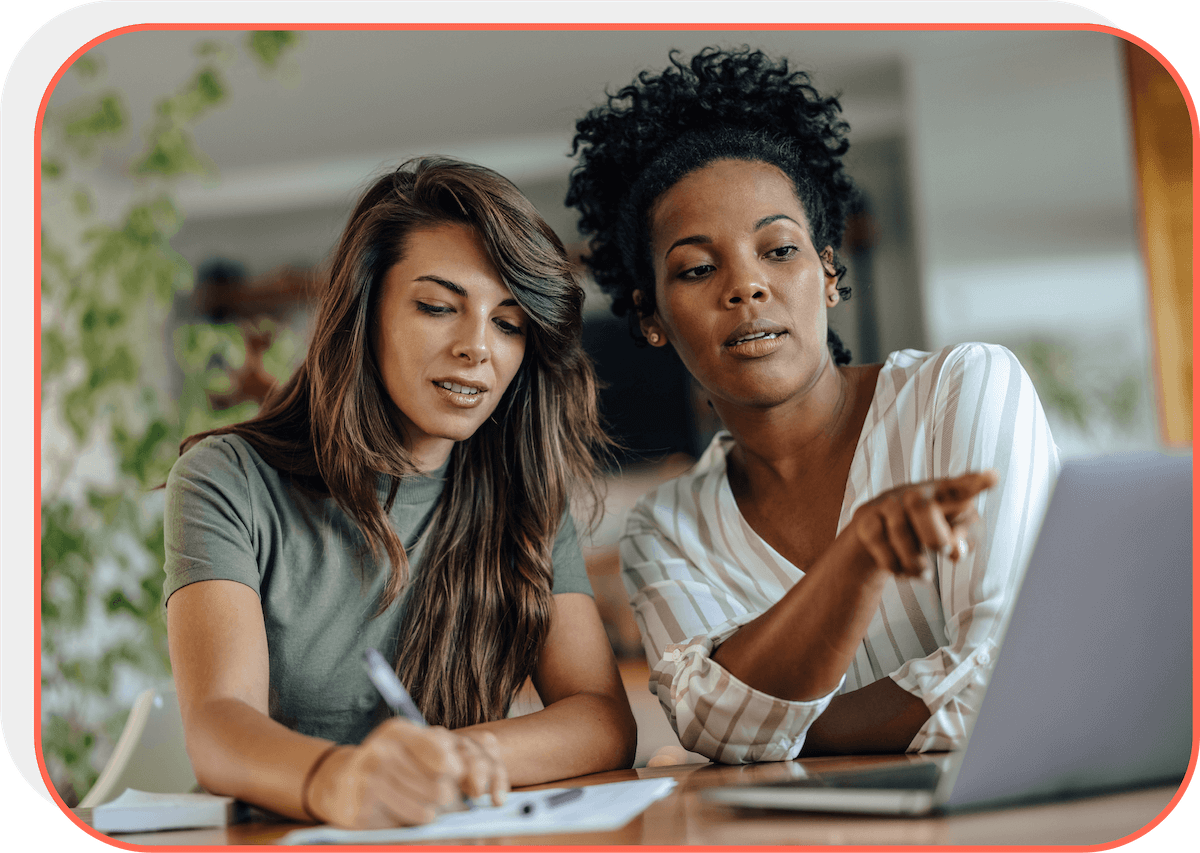
Marketing mix modeling might feel unfamiliar and counterintuitive at first, especially if you’re coming from a last-touch attribution mindset. But trusting the system—and understanding the depth of analysis behind its recommendations—will unlock new levels of efficiency and ROI for your campaigns.
Just as you wouldn’t choose the car with the smallest fuel tank for the quickest long road trip, you shouldn’t assume the lowest CPA channel is necessarily the best. MMM gives you the full picture, enabling you to make smarter, more holistic marketing decisions.
By leveraging AIM, you’re able to make data-driven, strategic choices that optimize your entire marketing mix—even if the recommendations initially surprise you!
Want a consultation on your current media mix? Get in touch with the AIM team.